table of contents
stay up to Date about neospectra
Introduction
New soybean varieties have been developed through genetic engineering, featuring increased oleic fatty acid. This mono-unsaturated fat is considered a healthier oil due to its lower saturated fat content, as well as its higher oxidative stability and longer shelf life compared to conventional soybean oils. This enhanced stability eliminates the need for hydrogenation, ensuring the absence of trans-fat—an important factor for the food industry, particularly with the ban on partially hydrogenated oils (Food and Drug Administration, 2015). The industry needs a quick on-spot analysis to detect the quality parameters of the receiving soybeans, especially to analyze high-value materials. Moreover, this capability should be refined to be applied in a controlled environment that provides extra accuracy to the analysis
Overview
Genetically modifying soybeans to enhance their oleic acid composition offers a practical approach to increasing the supply of high oleic vegetable oil, addressing current limitations in the market. The development of soybean varieties that accumulate high oleic oil while preserving protein quality represents a potential sustainable solution to meet future nutritional demands.
As the production of new specialty soybean oils, particularly high oleic variants, continues to rise, and regulations on GMO food ingredients become more stringent, effective monitoring of incoming soybeans is essential for food companies. Conventional methods for this purpose are often time-consuming, labor-intensive, and require skilled person- nel. Therefore, the identification of rapid and straightforward screening methods is crucial for ensuring the efficiency of food produc- tion.
The NeoSpectra Scanner, characterized by its compact size and cost-effectiveness, emerg- es as an ideal candidate for serving as a screening tool to differentiate GMO high oleic soybeans from conventional ones. Fur- thermore, its versatility allows for application in a controlled environment where sample preparation (such as grinding) is permissible, enhancing the accuracy of the parameters.
How NIR Works
NIR is a secondary analytical method that relies on the data coming from the reference laboratory (GC-FID), reference data, to build PLS regression with the spectra. Once it is calibrated, the PLS model enables predicting fatty acid composition using only the spectra generated by the NIR spectrometer. Results can then be obtained in less than 2 minutes by the instrument.
Experiment Design
NIR is a secondary analytical method that relies on the data coming from the reference laboratory (GC-FID), reference data, to build PLS regression with the spectra. Once it is calibrated, the PLS model enables predicting fatty acid composition using only the spectra generated by the NIR spectrometer. Results can then be obtained in less than 2 minutes by the instrument.
Sample Sets
A total of 88 samples were collected from4 different suppliers. Samples were conventional soybeans and higholeic soybeans. To ensure the performance of the models on difference units, a total of 17 instruments were used to collect spectra. The instruments were divided in 5 (Calibrationunits) and 12(Development kit) instruments. Finally, two whole soybean samples were collected at -20C, 4C and 20C to check the performance of the models exposed to extreme temperatures.
Reference methods
Gas Chromatography (FID) was used to analyze the fatty acid profile from the ether-extracted fat. Results are expressed in grams of fatty acid per 100g of sample.

Calibration and validation sets
Cross-validation was used to evaluate the model performance. Moreover, 2 samples were measured at 3 different temperatures to see the effect of the temperature on the predictions. This was done to ensure that the models for whole beans would perform well when they are in the field at negative and positive temperatures.
Measurement Conditions
• Setup: Diffuse reflection
• Spectral range: 1350 – 2550 nm
• Scan time: of 5s
• Resolution of 16nm at λ=1,550 nm
• Spot size = 10 mm2,
• Temperature: Room temperature
(* except 2 samples)
• Averaging: Each sample was measured 6 times for ground samples and 12 times for whole beans with the NeoSpectra Scanner and averaged for the analysis
Calibration Model Development
Partial least squares regression (PLS) models were constructed to establish the linear relationship between the spectra and composition, determined through laboratory chemical analysis. PLS is employed to reduce spectrum data, originally comprising 257 variables (wavelengths), into a limited number of latent variables (L.V.). This reduction in complexity aims to enhance the interpretability of the data.
The selection of latent variables is based on their correlation with the responses (soybean contents in this context), prioritizing those with high correlation.
Data analysis
The performance of the Partial Least Squares (PLS) model was evaluated using a cross-validation technique. This involved calculating the prediction error (root mean square of errors for all samples) and the coefficient of determination (R2CV) between predicted contents and the reference data obtained from chemical analysis. The cross-validation technique entails dividing the data into calibration and validation sets. The calibration set is utilized for training the PLS model, while the validation set is reserved for evaluating the model's performance.
In each iteration, the validation and calibration sets are combined, and a new portion of data is designated as the validation set. The process is then repeated, involving model training and validation on the updated sets. This iterative procedure continues until each sample has been represented at least once in the validation set, thereby providing a comprehensive assessment of the PLS model's predictive capabilities.
Results and Discussion
Results from the cross-validation are shown in Figure 1. In order to quantify the accuracy of the model, the following statistical characteristics are summarized in:
•R2: Determination coefficient. The closerto 1 the better.
•RMSE: Root Mean Square of the Error. Thesmaller the better.
•SEP – Standard Error of prediction. Thesmaller the better.
•Bias – mean difference between laborato- ry results and predicted values. The closerto 0 the better.
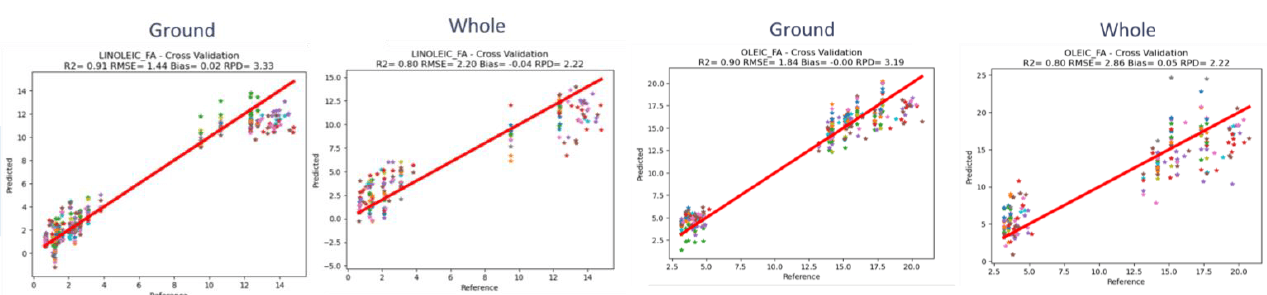
The findings presented in this study suggest that the NeoSpectra Scanner provides excellent results in predicting ground samples. Moreover, whole soybeans can be analyzed for a quick analysis providing a screening tool without sample preprocessing (Table 2).

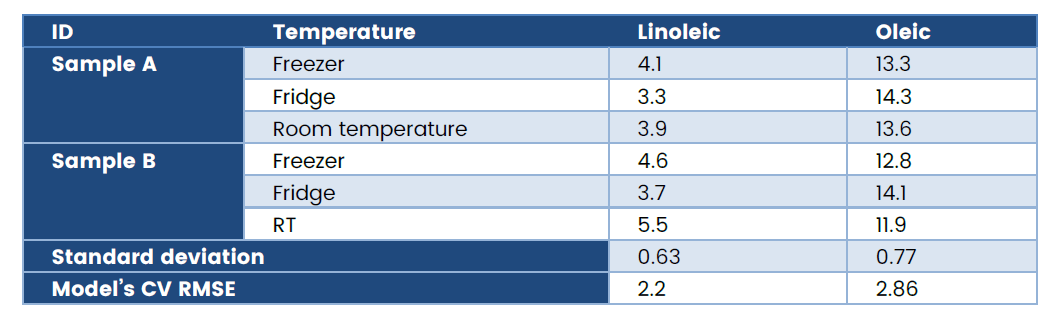
Conclusions
The NeoSpectra Scanner has demonstrated excellent performance in analyzing whole soybeans and ground soybeans, enabling on-field testing. Samples collected directly from the truck in the receiving area can be promptly analyzed, and the resulting data can be utilized to assess soybean quality. For an extra accuracy, same instrument can be used after grinding the sample. All the information is securely stored in the cloud, ensuring accessibility from anywhere in the world. This capability is applicable to both small scale and large corporations, offering a versatile solution that seamlessly integrates into various productions and farm setups.
Acknowledgment
This article was made possible by the contributions of Cumberland Valley Analytical Services (CVAS). Special acknowledgment goes to Heather Seibert and Ralph Ward for their valuable contributions and data.